Recent advancements in artificial intelligence have highlighted its potential to revolutionize neuroscience research. A groundbreaking study led by researchers from University College London (UCL) demonstrates that large language models (LLMs) can predict the outcomes of neuroscience studies more accurately than human experts. The study, published in Nature Human Behavior, found that LLMs achieved an 81% accuracy in predicting experimental results, compared to just 63% for neuroscientists.
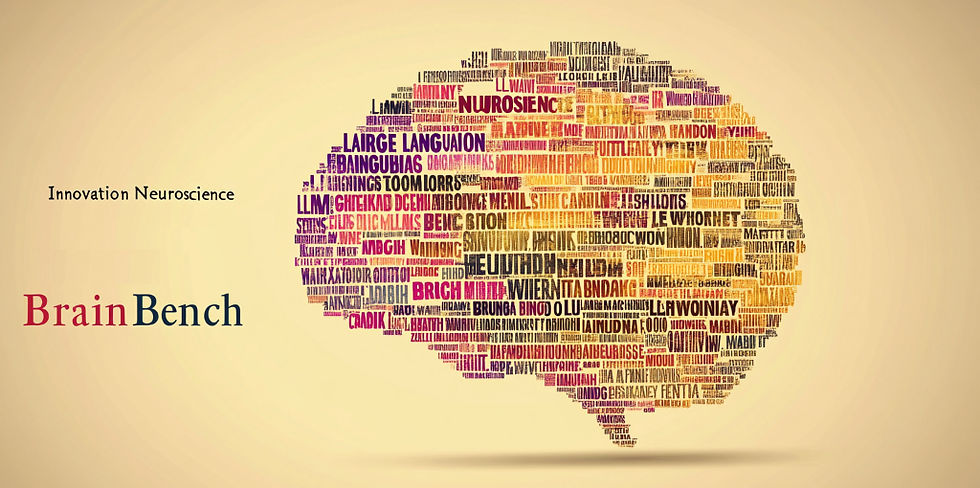
Understanding LLMs
Large Language Models (LLM) are a type of artificial intelligence programs that can process, understand, and generate human language. These deep learning systems are built on the transformer architecture, which includes an encoder and decoder that utilize self-attention mechanisms to understand the relationships between words and phrases in a sequence. Unlike earlier models like recurrent neural networks (RNNs), which process data step by step, transformers handle entire sequences of text in parallel. This parallel processing enables the efficient use of GPUs during training, drastically reducing the time needed to train these models.
Through self-learning, transformers can grasp fundamental aspects of language, including grammar and general knowledge. These models, which can have billions of parameters, are trained on massive datasets allowing them to process vast amounts of information from the internet. OpenAI's GPT-3 has 175 billion parameters and can generate natural, readable text. AI21 Labs' Jurassic-1 model has 178 billion parameters and a large vocabulary, while Cohere's Command model supports over 100 languages.
LLMs are highly versatile, capable of performing a wide range of tasks such as answering questions, summarizing texts, translating languages, and completing sentences. These models have the potential to change how we create content and interact with search engines and virtual assistants. A key element in how LLMs work is how they represent words through multi-dimensional vectors. Compared to earlier models which utilized numerical tables to represent words, the use of multi-dimensional vectors allows for the ability to group similar words with similar meanings together within a vector space. This solved the problem of previous models being unable to recognize how words relate to each other. With this, transformers can convert text into numerical representations through the encoder, allowing them to understand the context of words and their relationships, such as parts of speech or similar meanings. This understanding is then applied through the decoder, enabling LLMs to generate meaningful and contextually appropriate output.
LLMs in Scientific Prediction
One of the most exciting possibilities for LLMs lies not just in their ability to summarize and retrieve knowledge, but in their potential to predict future outcomes based on patterns in existing data. Dr. Ken Luo, lead author of the study from University College London, highlighted this very potential: “Since the advent of generative AI like ChatGPT, much research has focused on LLMs’ question-answering capabilities, showcasing their remarkable skill in summarizing knowledge from extensive training data. However, rather than emphasizing their backward-looking ability to retrieve past information, we explored whether LLMs could synthesize knowledge to predict future outcomes. Scientific progress often relies on trial and error, but each meticulous experiment demands time and resources. Even the most skilled researchers may overlook critical insights from the literature. Our work investigates whether LLMs can identify patterns across vast scientific texts and forecast outcomes of experiments.”
In this sense, LLMs could revolutionize not only how we analyze existing knowledge but how we predict new findings, offering invaluable insights into the future of scientific research.
Predicting Outcomes Through BrainBench
The study leveraged a tool called BrainBench, designed to test both AI models and human experts on their ability to distinguish between real and fabricated neuroscience study abstracts. The abstracts were paired in such a way that one described a legitimate research study, while the other presented a modified, but plausible, set of results. Researchers tested 15 different general-purpose LLMs, as well as 171 human neuroscience experts, all of whom had been screened for their qualifications.
Surprisingly, the LLMs outperformed the experts across the board, with average accuracy rates of 81% compared to the 63% accuracy achieved by human neuroscientists. Even when the study restricted the results to only the most experienced neuroscientists, the LLMs still held a clear advantage, with the highest-credentialed experts achieving only 66% accuracy. Not only did the LLMs outperform the experts, but the study also found that the more confidence the LLM had in its decision, the more likely they were to be accurate in their findings.
BrainGPT as a Specialized Model for Neuroscience
By adapting an existing LLM, the researchers were able to develop BrainGPT which is a specialized LLM trained specifically on neuroscience literature. BrainGPT outperformed its previous counterparts, reaching an impressive 86% accuracy in predicting neuroscience outcomes, suggesting that tailored AI models could further enhance research predictions.
This study has significant implications for the future of scientific research. It suggests that AI tools, like LLMs, could play a key role in designing experiments, predicting results, and ultimately accelerating the pace of scientific discovery. As AI continues to evolve, researchers could use models like BrainGPT to anticipate the likelihood of various outcomes based on existing scientific knowledge, leading to faster and more informed decision-making.
According to Professor Bradley Love of UCL stated “In light of our results, we suspect it won’t be long before scientists are using AI tools to design the most effective experiment for their question. While our study focused on neuroscience, our approach was universal and should successfully apply across all of science.”
References: